MLflow
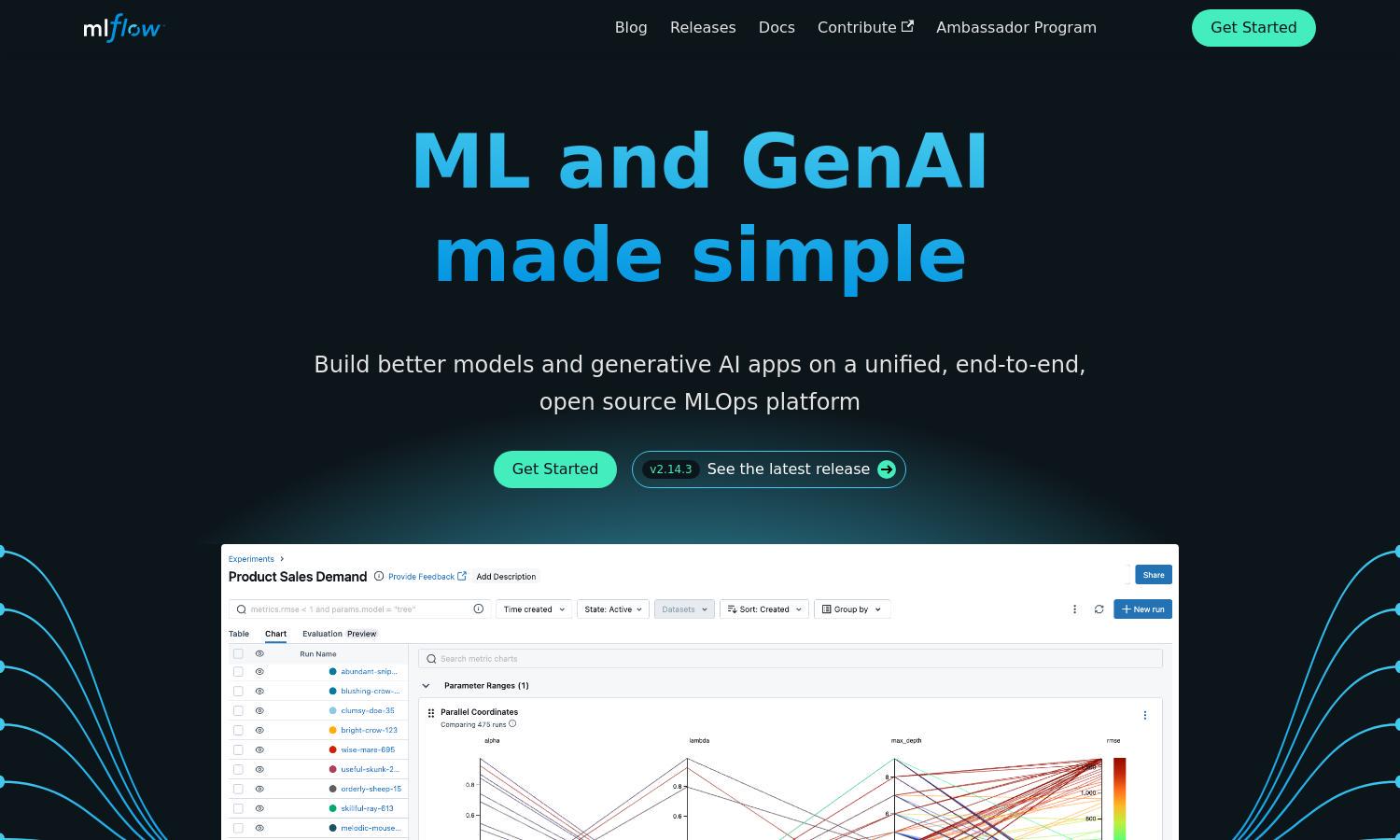
About MLflow
MLflow is a versatile open-source platform designed for data scientists and machine learning engineers. It streamlines the entire ML lifecycle—from experimentation to deployment—allowing users to track experiments, manage models, and deploy applications with unprecedented ease, ultimately enhancing productivity and workflow efficiency.
MLflow offers a free open-source platform with options for enterprise support. While basic features are accessible to all users, premium offerings provide enhanced functionality for managing complex ML workflows. Investing in premium tiers unlocks greater efficiencies and support for scaling operations.
The user interface of MLflow is designed for maximum efficiency, featuring an intuitive layout that simplifies navigation through its powerful tools. Users benefit from a streamlined experience that enables quick access to key functionalities, driving productivity and enhancing their workflow in machine learning projects.
How MLflow works
Users interact with MLflow by first signing up and onboarding, after which they can explore various features. The platform provides tools for experiment tracking, model management, and deployment. Users can easily log their experiments, visualize results, and handle model registries, ensuring a streamlined workflow throughout the machine learning lifecycle.
Key Features for MLflow
Experiment Tracking
MLflow's experiment tracking feature enables users to log and compare various runs seamlessly. This essential capability provides insights into different model performances, helping to optimize results and streamline the model development process, making MLflow indispensable for machine learning practitioners.
Model Registry
The Model Registry in MLflow allows users to centralize model management, promoting streamlined version control and deployment. This feature underscores MLflow's value, ensuring that users can efficiently track their models' lifecycle stages, enhancing collaboration and reducing deployment errors in production environments.
Deployment Integration
MLflow’s deployment integration feature simplifies the process of deploying models across various environments. By providing built-in support for diverse platforms and tools, MLflow ensures that users can effortlessly package and deploy their models, thus reducing the time from development to production.
You may also like:
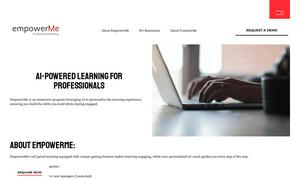
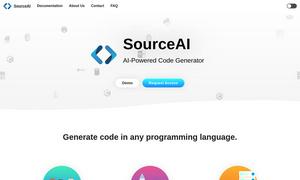